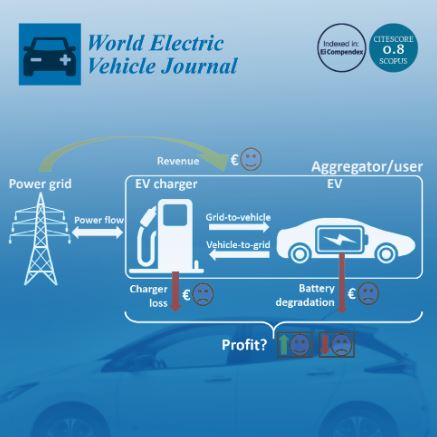
Improved Prediction of Total Energy Consumption and Feature Analysis in Electric Vehicles Using Machine Learning and Shapley Additive Explanations Method
Publication Year: 2021
Author(s): Pokharel S, Sah P, Ganta D
Abstract:
Electric vehicles (EVs) have emerged as the green energy alternative for conventional vehicles. EVs also appear to be the most promising choice for improving the fuel economy, but they still have significant disadvantages due to the limited driving range for the battery. While various governments promote EVs, people feel “range anxiety” because of their limited driving range or charge capacity. A limited number of charging stations are available, which results in a strong demand for predicting energy consumed by EVs. In this paper, machine learning (ML) models such as multiple linear regression (MLR), extreme gradient boosting (XGBoost), and support vector regression (SVR) were used to investigate the total energy consumption (TEC) by the EVs. The independent variables used for the study include changing real-life situations or external parameters, such as trip distance, tire type, driving style, power, odometer reading, EV model, city, motorway, country roads, air conditioning, and park heating. The authors compared the ML models’ performance along with the error analysis. A pairwise correlation study showed that trip distance has a high correlation coefficient (0.87) with TEC. XGBoost had better prediction accuracy (~92%) or R2 (0.92). Trip distance, power, heating, and odometer reading were the most important features influencing the TEC, identified using the shapley additive explanations method. The results demonstrate that predictive ML algorithms can assist drivers by providing insight into conditions that can influence the TEC of their EVs. The use of ML in predicting the TEC might be used to improve the performance of various models of EVs.
Source of Publication: World Electric Vehicle Journal
Vol/Issue: 12, 94: 1-10p.
DOI No.: 10.3390/wevj12030094 -
Country: India
Publisher/Organisation: MDPI
Rights: Creative Commons Attribution (CC BY) license (https:// creativecommons.org/licenses/by/ 4.0/)
URL:
https://www.mdpi.com/2032-6653/12/3/94/pdf
Theme: Research and Development | Subtheme: Software components/ICT
Related Documents
Journals
IEEE Transactions On Intelligent Transportation Systems
Published Year: 2000
Abstract:
The IEEE Transactions on Intelligent Transportation Systems published peer reviewed articles a... Read More
Journals
IEEE Intelligent Transportation Systems Magazine
Published Year: 2009
Abstract:
The IEEE Intelligent Transportation Systems Magazine (ITSM) is a quarterly publication. It pub... Read More
Journals
International Journal Of Automotive Technology And Management
Published Year: 2001
Abstract:
International Journal of Automotive Technology and Management is a quarterly publication. The... Read More